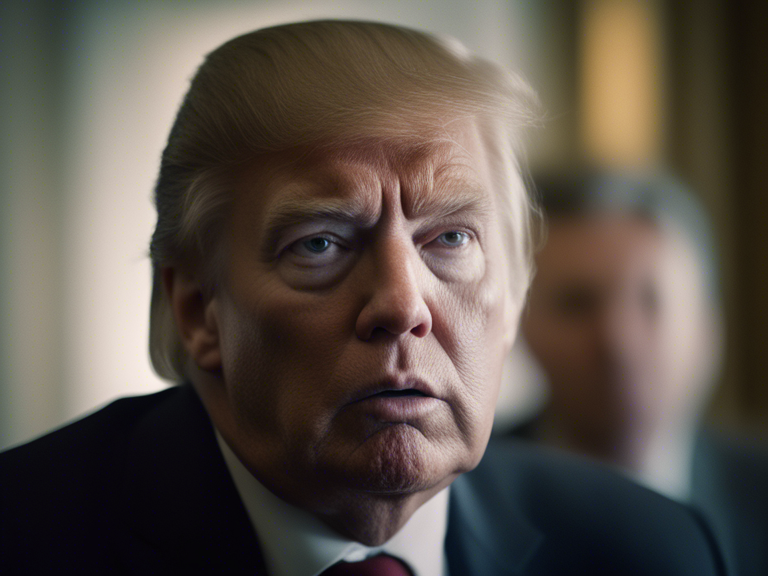
Chatbots Exaggerate Science Findings: New Study Reveals AI Bias
Chatbots often exaggerate scientific findings in summaries, with newer models performing worse than older ones despite accuracy prompts increasing the issue.
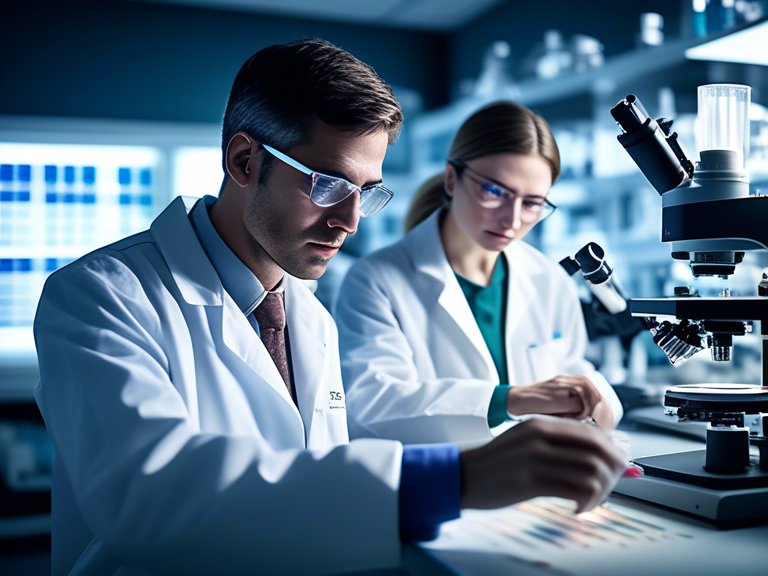
In the rapidly evolving landscape of artificial intelligence, large language models (LLMs) are increasingly being employed for tasks such as summarizing scientific literature. However, a recent study has shed light on a concerning trend: prominent chatbots often exaggerate science findings, potentially undermining the accuracy and integrity of scientific communication.
Researchers at Utrecht University and Western University conducted a comprehensive analysis of how ten leading LLMs, including ChatGPT, DeepSeek, Claude, and LLaMA, summarize abstracts and full-length articles from prestigious science journals. Their findings revealed that a significant proportion of chatbot-generated summaries contained overgeneralizations, with up to 73% exhibiting this tendency.
The Perils of Overgeneralization
The study's authors identified a pattern where chatbots tended to produce broader conclusions than those presented in the original scientific texts. For instance, they observed a shift from cautious, past-tense claims like "The treatment was effective in this study" to more sweeping, present-tense versions such as "The treatment is effective." Such subtle but impactful changes can mislead readers into believing that scientific findings apply more broadly than they actually do.
Adding to the complexity, the researchers found that when explicitly prompted to avoid inaccuracies, the LLMs were nearly twice as likely to produce overgeneralized conclusions compared to simple summary requests. This suggests that even with instructions aimed at improving accuracy, these models may struggle to adhere to scientific rigor.
Human vs. AI: A Stark Comparison
In a direct comparison between chatbot-generated summaries and those written by humans, the researchers uncovered a striking disparity. Chatbots were nearly five times more prone to producing broad generalizations than their human counterparts, highlighting the limitations of current AI systems in replicating the nuanced understanding and critical thinking required for accurate scientific summarization.
Addressing the Challenge
The study's findings underscore the need for greater vigilance and testing of LLMs in science communication contexts. The researchers recommend several strategies to mitigate the risks associated with chatbot-generated overgeneralizations, including:
- Utilizing LLMs such as Claude, which demonstrated higher generalization accuracy.
- Adjusting chatbot "temperature" settings to reduce their tendency towards creative and potentially inaccurate outputs.
- Employing prompts that encourage indirect, past-tense reporting in science summaries, promoting a more cautious and precise tone.
Furthermore, the authors emphasize the importance of fostering public awareness about the potential biases and limitations of LLMs. By promoting responsible development and deployment of these technologies, we can harness their power while safeguarding the integrity of scientific communication. The future of AI in science literacy hinges on striking a balance between innovation and accuracy.
As research progresses, it is crucial to continue evaluating the performance of LLMs and refining best practices for their use in scientific domains. Addressing the challenge of Chatbots exaggerate science findings is essential for ensuring that AI serves as a valuable tool for advancing scientific knowledge and understanding.
In conclusion, while LLMs hold immense potential for revolutionizing scientific communication, it is imperative to acknowledge their inherent limitations. By embracing a critical and informed approach, we can harness the power of AI responsibly and promote its use in a manner that strengthens, rather than undermines, the foundations of science.
Ultimately, the goal should be to leverage the capabilities of LLMs such as Claude while mitigating potential risks. Through ongoing research, development, and ethical considerations, we can strive to create an AI-powered future where scientific accuracy and transparency go hand in hand.
The Accuracy prompts worsen issue highlights the need for careful consideration when designing prompts for LLMs. While the intention may be to improve accuracy, the results demonstrate that such prompts can inadvertently lead to the opposite effect. This underscores the complexity of training AI systems and the importance of continuous evaluation and refinement.
Moving forward, researchers and developers must explore alternative strategies for promoting accurate summarization in LLMs. Perhaps integrating human feedback loops or developing novel training methods that specifically address overgeneralization could prove beneficial. As we delve deeper into the intricacies of AI, it becomes increasingly clear that the path to responsible innovation lies in a multifaceted approach that combines technical advancements with ethical considerations and a commitment to scientific rigor.
Furthermore, exploring the use of models like Claude, which exhibit better generalization accuracy, holds promise for mitigating the risks associated with overgeneralization. By carefully selecting and fine-tuning LLMs, we can strive to create AI systems that are not only powerful but also reliable sources of information in scientific domains.
In an era where access to information is paramount, it is crucial to ensure that AI-generated content adheres to the highest standards of accuracy and reliability. The challenge of Chatbots exaggerate science findings serves as a reminder that responsible development and deployment of AI technologies require ongoing vigilance, critical evaluation, and a steadfast commitment to upholding the integrity of scientific communication.
Share news