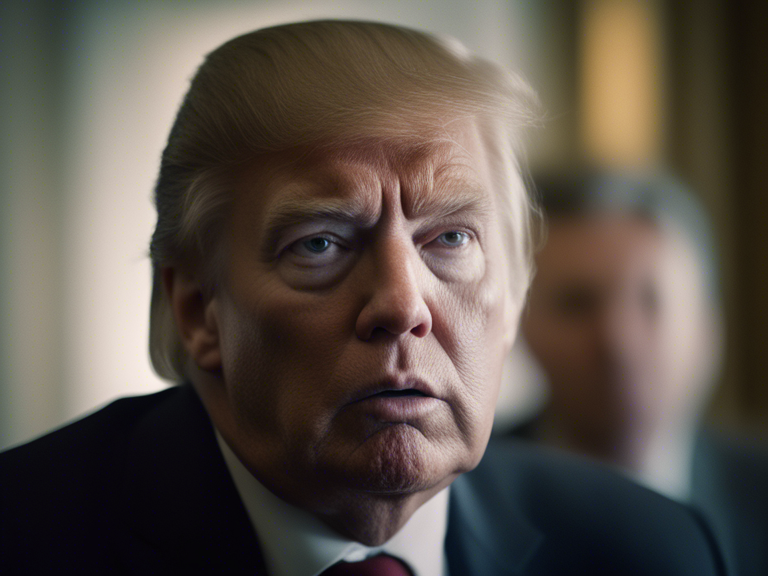
TinyAI Makes Big Strides: $30 Model Achieves Breakthrough Reasoning Abilities
Emerging research shows that smaller, more efficient AI models can achieve comparable reasoning abilities to larger, expensive models, challenging the industry focus on size.
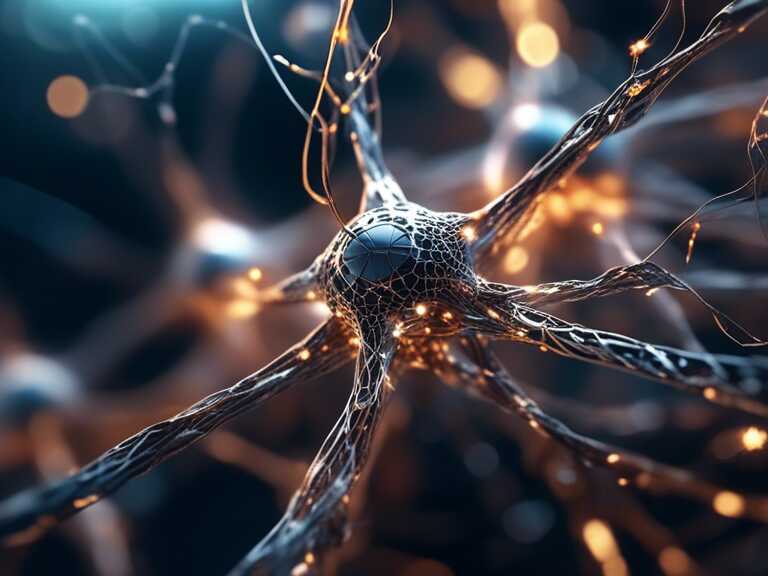
In the ever-evolving world of AI and technological advancements, the debut of DeepSeek's R1 generative AI large language model for a strikingly low $6 million has ignited debates and skepticism, especially amidst the multibillion-dollar investments from industry giants like Microsoft-backed OpenAI. As the financial stakes and expansion efforts in the AI market continue to make headlines, questions surrounding the sustainability and pace of growth in this sector have gripped the attention of analysts and investors alike.
Skepticism and Ambitious Plans in the AI Market
The cost-effectiveness of DeepSeek's generative AI model has raised eyebrows across the AI market, with the $6 million price tag standing in stark contrast to the hefty investments calculated in the billions made by major players such as OpenAI. Despite skepticism regarding DeepSeek's budgetary claims, OpenAI's impressive fortunes continue to attract substantial backing, currently eyeing a colossal $40 billion financing round at a potential valuation of $300 billion. Projected revenue for OpenAI is set to surge to $12.7 billion this year, further solidifying its position in the industry. Meanwhile, other key players like CoreWeave are looking to rejuvenate the IPO market, adding momentum to the AI stock offering trend.
Amidst growing concerns over the relentless pace of development and substantial financial commitments plaguing the AI industry, the so-called "Magnificent 7" tech stocks have faced challenges in the market year-to-date. Alibaba's co-founder, Joe Tsai, has even raised red flags over a potential AI bubble forming in the U.S., prompting a recalibration of expectations and strategies in the ongoing AI race.
Emerging Trends and Experimentation in the AI Market
While doubts persist regarding the pace and expenditure of the AI industry, rapid developments and significant investments remain a commonality. As the AI domain undergoes transformative changes, some U.S.-based entrepreneurs and researchers are accelerating their efforts in exploring budget-friendly routes to push the boundaries of large language model building. The UC Berkeley researchers, for instance, were at the forefront of reproducing a small-scale language model of DeepSeek's R1, accomplishing the feat for a mere $30 by leveraging cloud resources.
This project, spearheaded by campus graduate researcher Jiayi Pan, exhibited remarkable foresight by swiftly grasping the potential of DeepSeek's release. The team's endeavor was further galvanized by breakthroughs from OpenAI, which inspired them to investigate a new reasoning paradigm for AI models and their capacity to "think" before responding.
Redefining Costs and Capabilities in AI Modeling
Despite the strides made in reproducing DeepSeek's R1, the reported cost of $6 million for training its R1 was deemed prohibitive by the researchers, fueling their quest for cost-effective yet capable solutions. The genesis of the TinyZero project was rooted in the premise that reducing task complexity and model size could still elicit emergent reasoning behavior while substantially curtailing costs. Through experimentation, the team successfully demonstrated that even with a model as modest as 3B, the AI was capable of learning to reason about straightforward problems and adapt its approach to find better solutions—a pivotal achievement exemplifying the depth of reasoning behavior exhibited by prevalent AI models.
Share news